Similarity matrices and Clustering
Review paper relating FineSTRUCTURE/ChromoPainter to other approaches
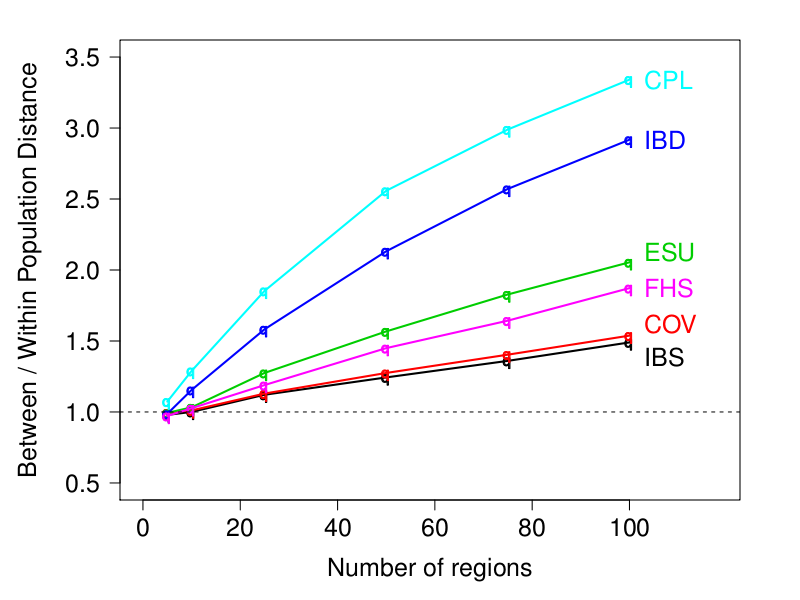
Caption: Measure of the information about population structure for different similarity matrices (higher is better). CPL is the ChromoPainter linked matrix, IBD is the FastIBD linked matrix, ESU is EIGENSTRAT unlinked matrix (and the ChromoPainter unlinked matrix), FHS is the FastPHASE linked matrix, COV is the covariance between individuals, and IBS is the Identity-By-State (and Allele Sharing Distance, ASD). The "Number of regions" on the x-axis measures how much sequence-style data is available - there are 2.5M SNPs in 100 regions.
Abstract
A large number of algorithms have been developed to identify population structure from genetic data. Recent results show that the information used by both model-based clustering methods and Principal Components Analysis can be summarised by a matrix of pairwise similarity measures between individuals. Similarity matrices have been constructed in a number of ways, usually treating markers as independent but differing in the weighting given to polymorphisms of different frequencies. Additionally, methods are now being developed that better exploit the power of genome data by taking linkage into account. We review several such matrices and evaluate their `information content'. A two-stage approach for population identification is to first construct a similarity matrix, and then perform clustering. We review a range of common clustering algorithms, and evaluate their performance through a simulation study. The clustering step can be performed either directly, or after using a dimension reduction technique such as Principal Components Analysis, which we find substantially improves the performance of most algorithms. Based on these results, we describe the population structure signal contained in each similarity matrix, finding that accounting for linkage leads to significant improvements for sequence data. We also perform a comparison on real data, where we find that population genetics models outperform generic clustering approaches, particularly in regards to robustness against features such as relatedness between individuals.Overview
In the PLoS 2012 paper we compare FineSTRUCTURE with the model-based approaches STRUCTURE and ADMIXTURE. However, there are many possible ways of acheiving similarity-based clustering that enjoy the same computational efficiencies of the ChromoPainter/FineSTRUCTURE approaches. These all work by forming a Similarity matrix (which can be defined many ways, one of which is ChromoPainter) and performing Clustering (e.g. FineSTRUCTURE, but other approaches exist).We examine a range of choices of similarity matrix, and show that the normalisation used by EIGENSTRAT and ChromoPainter is essential for extracting population signal when using an unlinked model. IBS and standard covariance are significantly worse. However, ChromoPainter and FastIBD can both produce similar quality matrices, which contain similar information about population structure but potentially different information about population history.
We compare many clustering algorithms to FineSTRUCTURE, particularly hierarchical methods (UPGMA), MCLUST and K-Means, and either using PCA/MDS to perform dimensionality reduction or working with the similarity matrix directly. We find that dimension reduction is essential for extracting population signal using these approaches. However, on real data where populations are small and the data is not clean, PCA gives a misleading signal and the populations found by these methods are not sufficiently robust.
The conclusion is that the ChromoPainter/FineSTRUCTURE pipeline is the preferred approach, being robust and using the information in the most efficient way currently available. However, methods could be developed that improve on these approaches but these must take into account the genealogical features of the data - generic approaches are unlikely to be sufficiently robust.